ETL Strategies & Pipelines have now become inevitable for cloud business needs. There are several ETL tools in the market ranging from open-source ones such as Airflow, Luigi, Azkaban, Oozie to enterprise solutions such as Azure Data Factory, AWS Glue, Alteryx, Fivetran, etc. But what makes the data pipelines to be Industry-ready and robust? The practices and the industrial standards that are put into the architecture and design of the pipelines do. In this article, we touch upon these aspects and characteristics of Enterprise-level practices that can be followed to make a robust data pipeline.
ETL-Alphabets
We will be explaining the ETL strategies, alphabetically as shown in the illustration below:
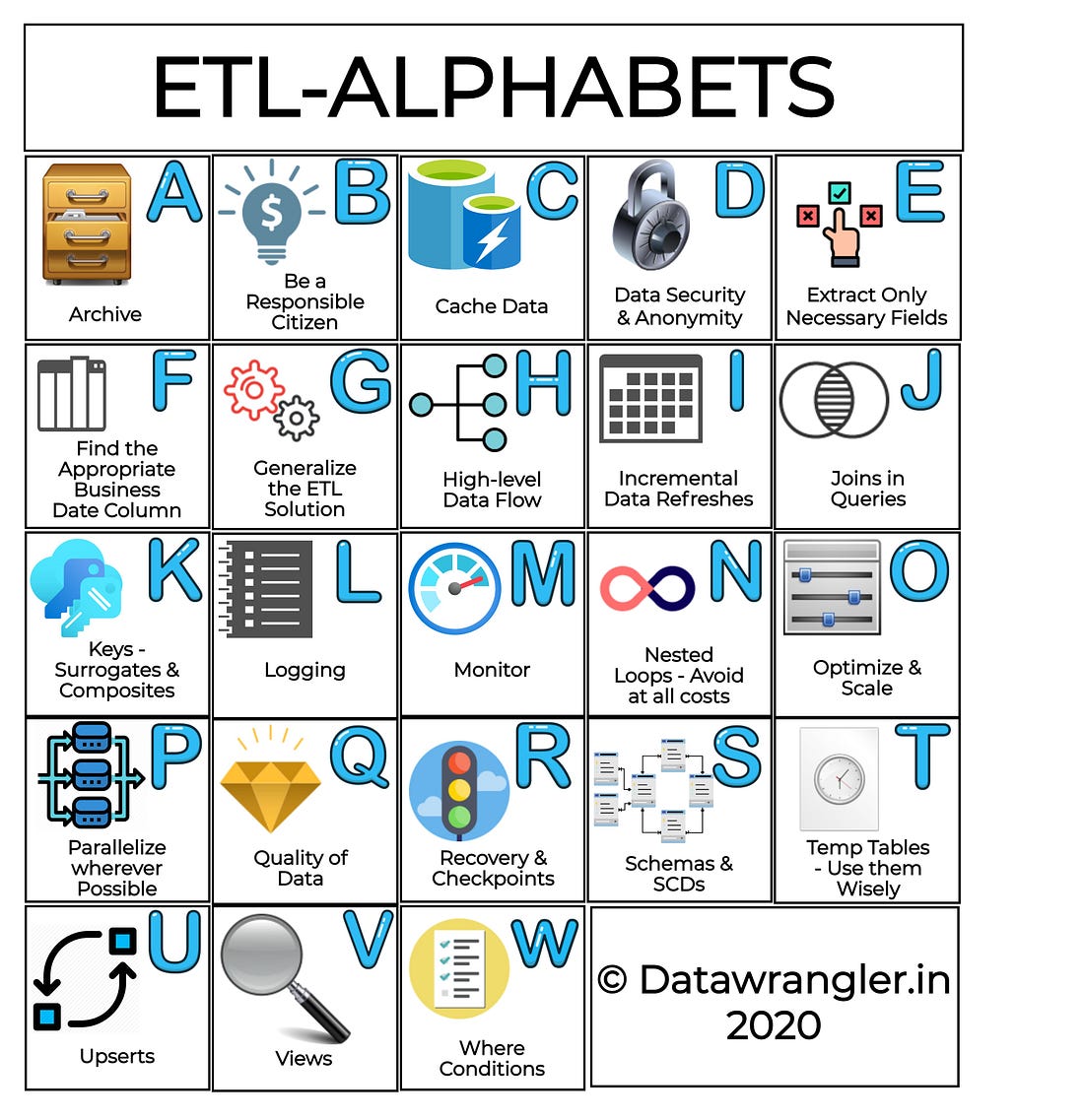
Archival Strategies
Archive older unused data, to keep the data warehouses clean and performant. By performing such cleanups, unused indexes can be cleared, and unused space can be retrieved.
Be a responsible citizen
- Select only those columns that are required for the analysis - While performing the extraction, select only the required columns. This will help in increasing the performance of the data warehouse and also, speeds up the extraction process.
- Power down resources when not in use. If you are using clusters for processing or databases for staging; make sure to power down the resources that are not in use.
Cache Data
Accessing a table more often? Why not cache that table for rapid usage? For example, Spark’s createOrReplaceTempView
function creates just a mere reference to the table whereas the saveAsTable()
stores the table to HDFS. This won’t make much of a difference for smaller datasets, yet could be a pivotal improvement while transforming larger datasets.
Data Security & Anonymity
Mask the sensitive information or better not to bring those to the target Data warehouse. Always perform your ETL practices over VPNs or secured groups. Always use a key vault service (Vault, Azure Key Vault, AWS Secrets Manager), etc. in case if you don’t have a Universal Authentication Identity Service Provider protected by individual Service Principals.
Extract Only Necessary Fields
You don’t always require a select * from table
. Select only those fields that are required from the source database tables. If you are wondering if you needed any columns in the future, many of the ETL solutions such as Azure Data Factory, provide options to facilitate schema drift. This could be a major game-changer in terms of the performance and efficiency of the ETL processes.
Find the Appropriate Business Date Column
Identifying the fields is one, and identifying the business date columns is another. The business date columns are essential since the data lake timestamp columns are not always reliable. These columns are essential for performing incremental changes to your data warehouse.
Generalize the ETL solution
The ETL solution that you create should be able to accommodate changes dynamically. Changes shouldn’t bring about havoc, rework, or major code changes. Make sure to bring in a lot of dynamic parameters and configurable placeholders such as File Location, File System Location, Logging Location, Name of Facts, Dimensions, Staging Tables, etc.
High-Level Data flow
Keep your ETL Pipelines and the corresponding data flows, as simple as possible. Plan before you start, draft a blueprint of what your pipeline is about to accomplish. This helps in figuring out those activities that are tricky and are prone to failures, such as processing at the staging layer and copy to the destination data warehouse.
Incremental Data Refreshes
Incremental data refreshes are nothing but bringing in the data that has changed or newly inserted into the Data Lake. Business date stamps can be very helpful while performing incremental data refreshes. Incremental data refreshes can be performed wherever it's applicable since performing a complete refresh of the data for the whole year can be expensive if done frequently.
Joins in Queries
Joins in queries are fine, as long as you know what you are doing and the query plan is under check. Make sure to check for the conditions used to join the query, filter the results even before you could join, if possible.
Keys-Surrogates & Composites
Surrogate Keys are columns that are added to the data warehouse tables to ensure uniqueness in the destination tables. These keys are essential to perform an upsert operation (insert and update if the insert fails) on the destination tables. The choice of columns is based on the business context and usually, these keys are framed as an SHA encryption of multiple columns combined using a delimiter.
E.g.: In a fact table representing details about the users purchasing products: Product_id, Order_timestamp, and Customer_id, Delivery_date uniqueness can be achieved for each entry by creating a surrogate key like SHA(Product_id, ‘|’, Order_timestamp, ‘|’, Customer_id). In case, there’s an update in the Delivery_date, the newer date can be updated based on the surrogate key in the Data warehouse.
While querying the data warehouse, it’s a good practice to check the query plan, if the indexes are properly used. If not, the required indexes can be created. For queries utilizing multiple columns, these can be recognized and a composite key combining these columns can be created for easier access.
Logging
Logging helps to track down the pesky ETL run failures. If the ETL application provides logging out of the box, no external logging is required. If not, it’s highly essential to have a custom script that does the logging.
Monitor
Mechanisms to monitor the ETL processes need to be in place. For example, Azure Data Factory and Airflow has some really good interfaces to track the failures. In addition to this, mechanisms such as e-mail alerts and slack notifications can be really helpful. In short, a holistic view of the pipeline’s health needs to be available to monitor at all times.
Nested Loops-Avoid at all costs
In most situations, the memory leaks observed in the ETL jobs are due to the Join Strategies. This can be found in the Query Plans. Depending on the database and processing engines, the strategy may vary.
For example: In Spark, the join strategy can be rated from ‘worst’ to ‘best’ as follows:
Broadcast nested loop join (worst) > Cartesian Product > sort-merge join > Hash Joins (best)
As noticed above nested loop join is chosen to be the last resort. A nested loop join strategy looks like this:
for each_row in relation_1:
for each_row in relation_2:
# join condition gets executed`
An n squared complexity is not something you would want to experience on a large dataset.
Optimize & Scale
ETL Pipelines can be optimized by finding the right time window to execute the pipeline. For example, while scheduling a pipeline to extract the data from the production database, the production business hours need to be taken into consideration so that, the transactional queries of the business applications are not hindered. Choosing the right choice of tools, processing clusters, and strategies can help scale the pipeline. For example, the choice of the clusters for processing can be decided by calculating the query runtime statistics and volume of the rows extracted.
Parallelize wherever possible
Parallel processing is always efficient than sequential processing. Big data storage solutions and non-columnar destinations work parallel in nature. Higher versions of relational databases such as Postgres has the ability to parallelize the queries too. The individual processes of ETL can also be parallelized if the tool provides a solution.
Quality of Data
The source can be in any form, i.e.) the data in data lakes can be in varied forms. However, the target data warehouses should always have clean data. The ETL scripts need to make sure that the data written to the destination needs to be of high quality. The quality parameters include precision in floats, prevent varchar overflows, split columns based on the present delimiters (‘|’, ‘,’, ‘\t’), use the appropriate compression formats, etc.
Recovery & Checkpoints
The ETL Pipelines are to be designed in a pessimistic way, by asking the ‘what-if’ questions. Assume that there’s an outage in the source data lake/datastore; Will the pipeline be capable enough to pause and resume or perhaps, recover or restore from the failed checkpoint? By designing such fail-safe strategies, the pipelines can be robust.
Schemas & SCDs
Warehouse and ETL designs are purely driven by the business requirements and the Engineers need to ask the right questions to design the pipeline and BI requirements that the business needs. Schema & Slowly Changing Dimensions are the major steering components for the warehouse design. The schemas can be Star schemas or Snow Flake schemas depending on how fast the schema evolves.
- Star Schemas are constant and each dimension is represented only by a one-dimensional table
- Snow Flake Schemas are a lot more granular compared to the Star Schemas where dimension tables are normalized further, split into multiple tables.
In addition to this, depending on the necessity to store the historic information of the facts or dimensions, the choice needs to be made between SCD1 or SCD2.
- SCD1 is the activity of updating the existing rows if the key matches.
- SCD2 is the activity where the older data is preserved/invalidated and inserts the newer rows, promoting them to be the latest.
Temp Tables-Use them Wisely
As mentioned in the ‘Cache Data’, temporary tables are just a mere representation of the physical tables. They are accessible only to the executed cluster, available until the cluster runs, and are slow for operations since it is neither in memory nor persisted. True to its name, these temp tables are destroyed upon the termination of the cluster.
Upserts
As mentioned in the section ‘Schemas & SCDs’, SCD1 will depend on the unique key based on which the upsert operation is done. Upserts are nothing but Inserts and Updates on conflict. Here, the conflict refers to the inability to insert since the key already exists, which will in turn lead to an upsert operation.
Views
Creating views on top of complex queries can be very helpful at times when the results of the query needed to be computed and used only a minimal number of times. If it’s too expensive to store, views can come in quite handy.
Where Conditions
While performing the transformations, selecting only the bucket of data that is required for the processing can save a large amount of computing time. The ‘where’ clauses can help in achieving this, especially during the incremental refreshes.